Unlocking the Power of Image Labeling Tools for Object Detection
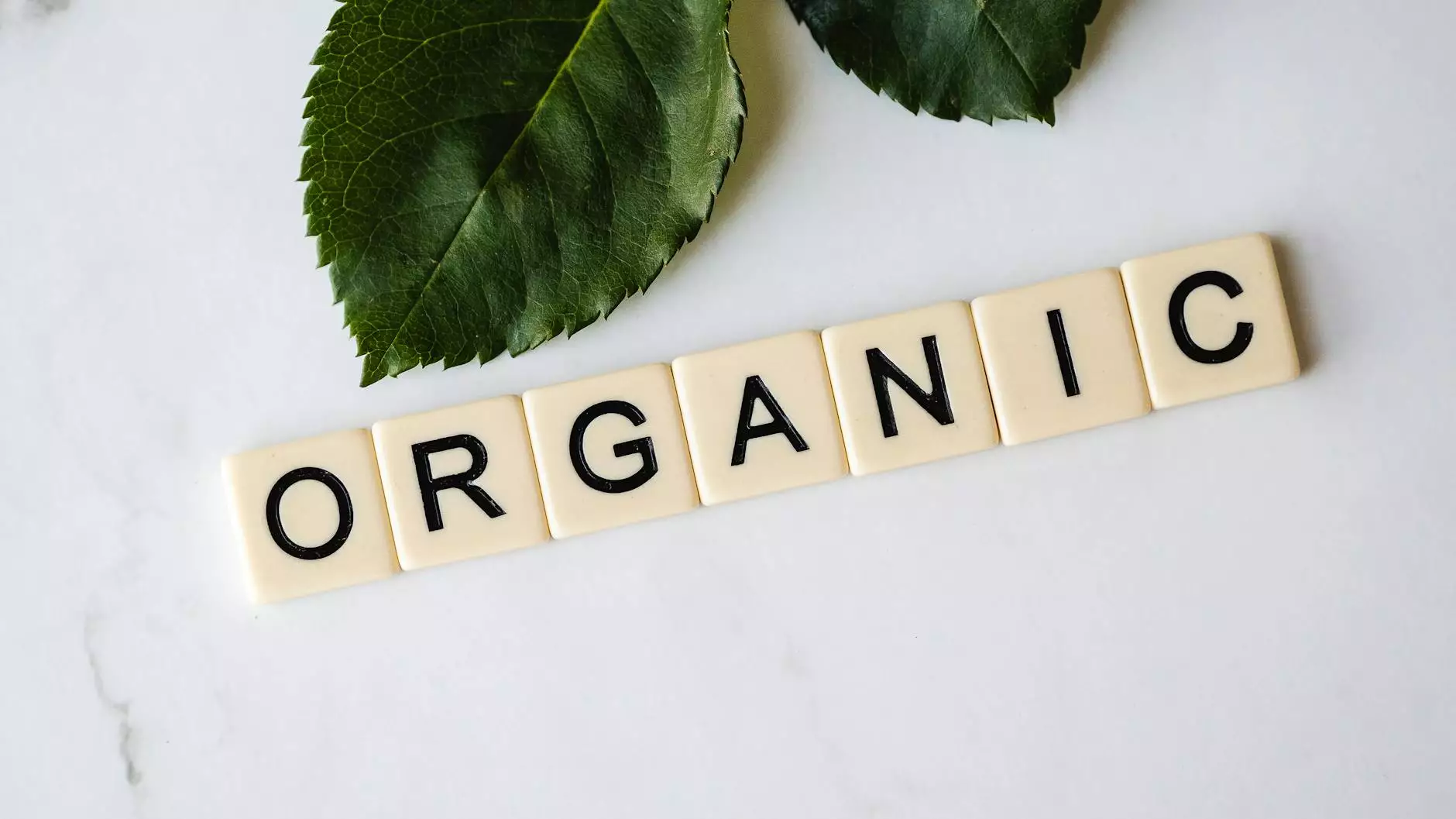
In the rapidly evolving landscape of artificial intelligence (AI) and machine learning (ML), the demand for precise and efficient data annotation has never been greater. Businesses across various sectors are seeking robust solutions to classify, label, and interpret data effectively. At the forefront of this technological revolution is the image labeling tool for object detection, a game changer for data annotation. In this comprehensive guide, we delve deep into the essential role these tools play, their benefits, and how they can optimize your data annotation processes.
Understanding Image Labeling in Object Detection
Image labeling is a crucial step in training machine learning models, particularly those that perform object detection. Object detection involves identifying and locating objects within an image using bounding boxes, segmentation, and classification. This requires meticulously labeled training data, making the annotation process vital for the development of accurate AI systems.
Without high-quality annotated data, no amount of sophisticated algorithms will yield effective results. Thus, businesses must utilize advanced image labeling tools that facilitate efficiency and accuracy in their projects.
Why Use an Image Labeling Tool?
Employing a dedicated image labeling tool can transform the data annotation process for organizations. Here are several reasons why investing in these tools is paramount:
- Increased Accuracy: Automated labeling tools reduce human errors, ensuring that the data is accurately labeled, which is essential for successful model training.
- Enhanced Efficiency: These tools significantly speed up the annotation process by automating repetitive tasks, allowing teams to focus on more complex aspects of data preparation.
- Scalability: A solid image labeling tool can easily scale to accommodate larger datasets, making it ideal for projects with vast amounts of visual data.
- User-Friendly Interfaces: Many tools are designed with intuitive interfaces that simplify the labeling process, making them accessible to users with varying technical skills.
- Integration Capabilities: Advanced labeling tools can often integrate seamlessly with existing workflows and machine learning environments, providing a cohesive operational experience.
Key Features of a Quality Image Labeling Tool
When selecting an image labeling tool for object detection, it is essential to consider a range of features that enhance usability and effectiveness. Here are some key functionalities to look for:
- Multi-Labeling Capability: Tools should support the ability to label multiple objects within a single image, accommodating complex scenes with overlapping items.
- Various Annotation Types: Look for a tool that supports a variety of annotation types such as bounding boxes, polygons, line segmentation, and point annotations to suit different project requirements.
- Collaboration Features: Many projects require input from multiple team members. Tools that offer collaborative features make it easier for teams to work together efficiently.
- Quality Control Mechanisms: Effective tools should provide ways to monitor and ensure label accuracy through review processes or audit trails.
- Export Compatibility: The ability to export labeled data in various formats is crucial for seamless integration with machine learning frameworks.
The Impact of Image Labeling on Business Outcomes
Utilizing an effective image labeling tool for object detection not only streamlines the annotation process but also significantly impacts overall business performance. Here are a few ways in which these tools can enhance business outcomes:
1. Enhanced Product Development
With accurate image labeling, organizations can develop advanced products such as autonomous vehicles, intelligent surveillance systems, and smart retail solutions. By ensuring that the trained models are based on high-quality, labeled datasets, businesses can drive innovation and stay ahead of the competition.
2. Improved Customer Experiences
In sectors like e-commerce or healthcare, accurate image recognition can lead to better customer experiences. For example, in e-commerce, personalized product recommendations are enhanced through effective image search and categorization, which is driven by precise labeling.
3. Cost Efficiency
Automating the annotation process saves time and reduces labor costs associated with manual labeling. Businesses that adopt efficient labeling tools can allocate resources to other critical areas, resulting in a more streamlined operation.
4. Better Compliance and Risk Management
High-quality image labeling can also improve compliance with regulatory standards, as having accurately labeled data helps organizations meet legal requirements in industries such as finance and healthcare.
Choosing the Right Image Labeling Tool
With numerous options available, selecting the proper image labeling tool for object detection can be overwhelming. Here are some critical considerations to help guide your decision:
- Assess Your Needs: Determine your specific project needs, such as the volume of images, types of objects to label, and required annotation styles.
- Evaluate Ease of Use: Opt for tools with user-friendly interfaces that facilitate quick adoption by your team.
- Check Customer Support: Reliable customer support is crucial for resolution of any issues that may arise during the annotation process.
- Look for Customization Options: Depending on your unique requirements, you may need a tool that allows for customization to better suit your workflow.
- Request a Demo: Whenever possible, request a demo or trial of the tool to evaluate its functionalities firsthand before making a purchase decision.
The Future of Image Labeling in AI
As AI and machine learning technologies continue to advance, the role of image labeling will only become more critical. Future developments may include:
- AI-Assisted Labeling: Leveraging machine learning algorithms to automate parts of the labeling process, thus reducing the time required for manual interventions.
- Real-Time Collaboration: Tools may evolve to facilitate real-time collaboration among annotators spread across different geographical locations.
- Enhanced Quality Control: Improved algorithms for validating label accuracy, ensuring higher quality datasets for training machine learning models.
Conclusion
The integration of an image labeling tool for object detection into your data annotation process can significantly improve the efficiency and accuracy of your machine learning initiatives. By selecting the right tool that meets your unique requirements, you can enhance your business operations, drive innovation, and deliver superior products and services in an increasingly competitive marketplace. As technology continues to evolve, staying ahead of the curve with advanced image labeling solutions is essential for any organization aiming to leverage the power of AI effectively.