The Role of Agriculture Datasets in Machine Learning: A Comprehensive Guide
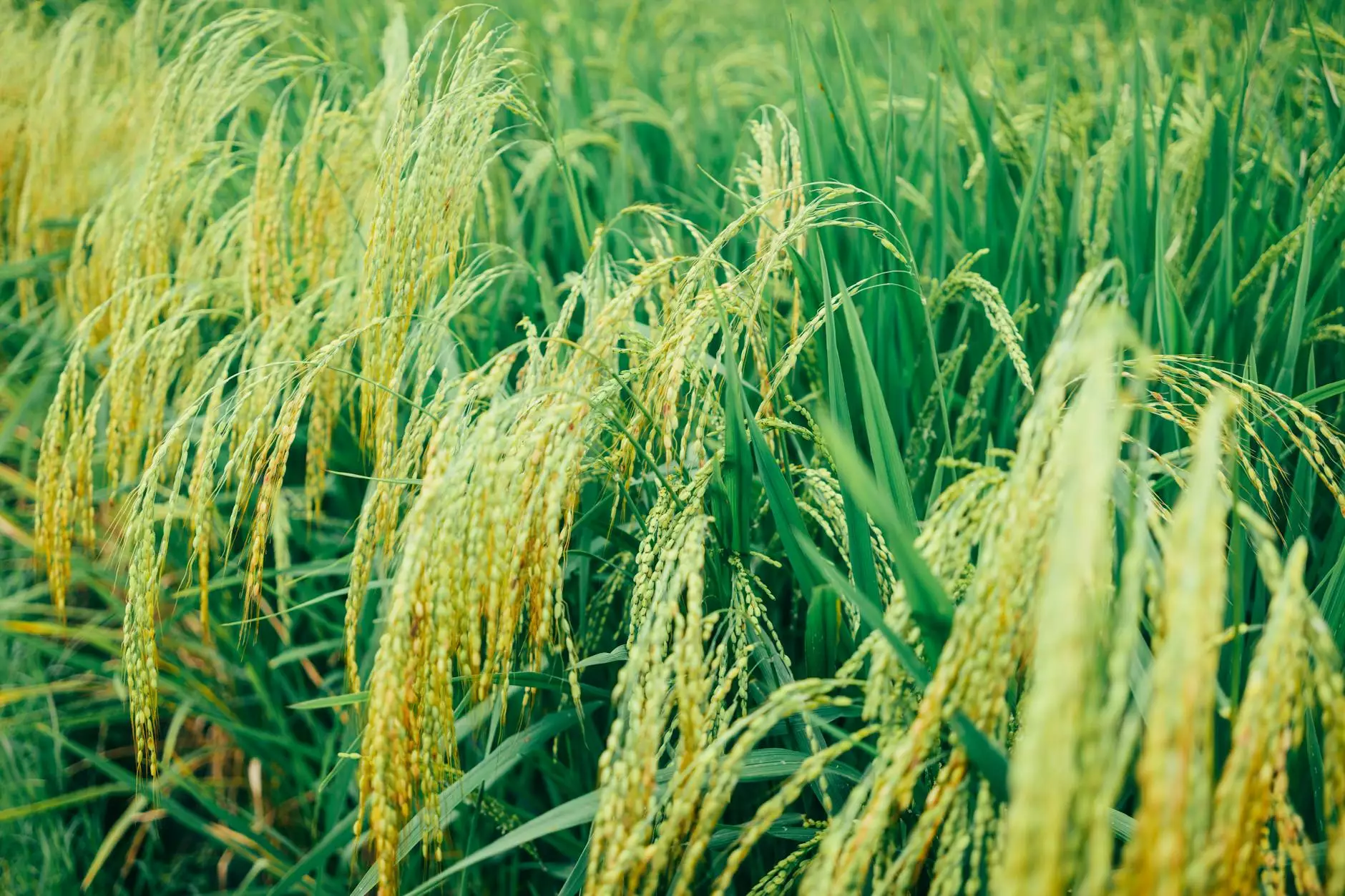
In today's world, technology is intertwined with every facet of human life, and agriculture is no exception. With the emergence of big data, particularly in the context of agriculture datasets for machine learning, the agricultural sector has begun to witness remarkable transformations. This article delves into the importance of these datasets, their applications, and the future they promise for the agricultural industry.
Understanding Agriculture Datasets
Agriculture datasets encompass a broad range of data points related to farming, crop yield, soil health, and environmental conditions. These datasets are critical for machine learning applications aimed at improving crop production, pest control, and resource management.
Types of Agriculture Datasets
Agriculture datasets can be categorized into several types, including:
- Soil Datasets: These include information on soil types, nutrients present, pH levels, and moisture content.
- Weather Datasets: Data capturing temperature, rainfall, humidity, and sunlight hours that affect crop growth.
- Crop Datasets: Information on different crops, their growth cycles, yield predictions, and disease susceptibility.
- Market Datasets: Data related to the prices of agricultural products, demand and supply trends, and global market insights.
- Pest and Disease Datasets: Information on pest prevalence, disease outbreaks, and treatment effectiveness.
The Importance of Agriculture Datasets for Machine Learning
Machine learning relies heavily on data to identify patterns and make predictions. In agriculture, the integration of machine learning with vast datasets offers unprecedented opportunities for efficiency and effectiveness.
1. Enhancing Crop Yield
One of the most significant benefits of utilizing agriculture datasets for machine learning is the enhancement of crop yield. By analyzing historical yield data alongside current environmental conditions, machine learning models can predict the most effective planting times, optimal crop varieties, and necessary agricultural practices to maximize harvests.
2. Predictive Analytics
Predictive analytics powered by machine learning allows farmers to forecast yields based on various inputs. For instance, by examining weather patterns and soil health data, farmers can make informed decisions that lead to better resource allocation and reduced waste.
3. Precision Agriculture
Precision agriculture leverages advanced technologies and datasets to monitor and manage field variability in crops. This approach helps in optimizing inputs like water, fertilizers, and pesticides, leading to increased financial efficiency and environmental sustainability.
4. Early Pest and Disease Detection
Agricultural datasets can also provide essential insights to predict pest invasions and disease outbreaks before they escalate. Machine learning algorithms can analyze trends and patterns in pest movements and disease conditions, allowing for proactive measures to protect crops.
5. Climate Adaptation
With the reality of climate change, agricultural practices need to adapt accordingly. Machine learning models can analyze climate data to understand potential impacts on agriculture, helping farmers to implement adaptive strategies such as crop rotation and diversification.
Collecting High-Quality Agriculture Datasets
For machine learning models to yield accurate results, high-quality datasets are crucial. Here are some critical factors in collecting effective agriculture datasets:
1. Data Relevance
Ensure that the data collected is relevant to the specific crops or farming practices being analyzed. Collecting data for multiple variables will provide a holistic view, thus improving model efficiency.
2. Data Accuracy
Incorrect data can lead to misleading results. It is vital to use reliable sources and validation methods when gathering data to maintain accuracy.
3. Timeliness
Data should be collected regularly to ensure that machine learning models can adapt to changing conditions. Timely data enhances predictive power and overall effectiveness in agricultural practices.
4. Data Integration
Combining datasets from various sources can unveil deeper insights. Integrating soil, weather, and crop data can lead to comprehensive models capable of addressing complex agricultural challenges.
Case Studies: Successful Applications of Machine Learning in Agriculture
Many businesses and organizations have begun utilizing machine learning and agriculture datasets to revolutionize farming practices. Here are a few notable examples:
1. John Deere
John Deere, a renowned agricultural machinery manufacturer, employs machine learning algorithms to analyze vast amounts of farming data collected through their equipment. This data helps farmers make informed decisions about planting and harvesting, improving yield and reducing costs.
2. AgroStar
AgroStar, an Indian agritech startup, leverages machine learning to provide farmers with insights derived from weather patterns, soil conditions, and pest data. Their platform helps farmers optimize their operations and improve crop outputs.
3. Climate Corporation
The Climate Corporation offers farmers data-driven decisions by analyzing weather data and historical crop yields. Their machine learning models predict harvests accurately, allowing farmers to plan better for market fluctuations.
The Future of Agriculture Datasets and Machine Learning
As technology continues to evolve, the future of agriculture datasets combined with machine learning looks promising. Here are some anticipated trends:
1. Increased Accessibility of Data
With advancements in technology, agriculture datasets will become more accessible. Open data initiatives will allow farmers, researchers, and policymakers to leverage data for better decision-making.
2. IoT Integration
The integration of the Internet of Things (IoT) in agriculture will facilitate real-time data collection. Devices such as soil sensors, weather stations, and drones equipped with cameras will gather data continuously, enhancing machine learning model accuracy.
3. Enhanced Decision Support Systems
Future machine learning applications will empower farmers with comprehensive decision support systems. With advanced analytics models, farmers can simulate various scenarios, leading to better planning and operational efficiency.
4. Focus on Sustainable Practices
Machine learning combined with agriculture datasets will emphasize sustainable agricultural practices. As the focus on eco-friendly farming increases, data-driven insights will help minimize the environmental impact of agriculture.
Conclusion
In conclusion, the integration of agriculture datasets for machine learning is set to revolutionize the agricultural landscape. With predictive analytics, precision farming, and the ability to make informed decisions, the agricultural sector stands to gain significantly. In a world where food security is paramount, leveraging data intelligently can lead to enhanced productivity and sustainability in agriculture.
As farmers and agricultural businesses become more data-driven, embracing these technologies and methodologies will be crucial to ensuring a sustainable and efficient future for farming.
agriculture dataset for machine learning